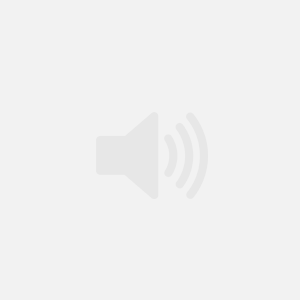
Episode Description
In this episode, Tim Hutchinson, co-founder and CEO, shares his journey from studying zoology to leading SkillsGPT, a pioneering generative AI business. Tim discusses how SkillsGPT is helping organisations adopt AI strategically, enabling them to adapt to change and gain a competitive advantage.
Tim provides examples of AI’s current and potential impact on work, emphasising the need for a ‘lifelong learning’ approach for all employees. He offers listeners a thoughtful perspective on ethical considerations, how AI is reshaping the workforce and how successful AI adoption should be led “from the boardroom, not the server room.”
Tim illustrates SkillsGPT’s role in helping 75,000 Australian regional SMEs adopt AI through the Australian Regional AI Network (ARAIN), a Federal Government-supported initiative. Focused on SMEs in agriculture, forestry, fisheries, and clean energy, ARAIN and SkillsGPT guide successful AI adoption with free access to tools, education, and insights. For FREE early access to ARAIN’s AI adoption resources, register at arain.com.au
The ARAIN resources offer value to all, not only regional SMEs.
About Tim Hutchinson and SkillsGPT
Connect with Tim on LinkedIn: https://www.linkedin.com/in/hutchinsontim/
Website: www.skillsgpt.ai
SkillsGPT is a full-service Artificial Intelligence studio, designing, delivering, and managing enterprise-grade AI solutions. Supported by Microsoft and InnovateGPT – Australia’s largest ecosystem of generative AI companies – SkillsGPT drives strategic AI adoption.
Beyond solution-building, SkillsGPT is ahead in educating organisations and their leaders to think strategically about AI. Through ‘AI Mindshift’ education and mentoring services, SkillsGPT establishes foundational AI knowledge and a mindset to adopt AI purposefully, ensuring sustainable success by aligning AI with organisational goals. Learn more at www.skillsgpt.ai
Points of Interest
6:23 Tim Hutchinson’s career in recruitment
11:14 How Tim got started in AI
17:51 Adopting AI
21:24 Using data with AI
22:38 Skills GPT
30:19 Potential security risks with AI
33:10 Examples of AI helping a business transform
39:17 Will AI take out jobs
41:56 Why you need to educate yourself on AI
46:27 Who are the winners
50:47 Advice for organisations wondering about AI
Transcript
Note: This has been automatically transcribed so is likely to have errors! It may however help you navigate the points of interest.
Michael McGrath 00:10
Welcome to the troubleshooters podcast with me, your host, Mike McGrath, now today’s guest, Tim Hutchinson is the co founder and CEO of Skills GPT. Tim started out in zoology, before falling into a career in recruitment, and then recently moving into the emerging world of AI. Tim now helps organizations harness the potential of generative AI to transform their organizations. So sit back as I seek to uncover fact from fiction in the new world of AI. Now a quick shout out to our sponsors, Oasis partners, corporate advisors with a practical bias. They may not know much about AI, but they do know a fair bit about getting a deal done. So if you’re considering an exit, talk to the team at Oasis. 500 deals done and counting. So Tim Hutchinson, welcome to the troubleshooters podcast.
Tim Hutchinson 01:03
Thank you, Mike, glad to be here.
Michael McGrath 01:04
No look, it’s great. And I know we’ve known each other for a little while, but when I bumped into your recent I thought we must get you in to have a chat about AI, because I’m sure a lot of our listeners who are business owners and people in corporate hearing lots of things about AI and generative AI and what it is and what it isn’t. And I thought you being, you being an expert, in fact, CEO of Skills GPT, I thought you might be a good guy, a good go to guy to sort of try and unpack, let’s hope AI So, yeah, welcome.
Tim Hutchinson 01:35
I can certainly share my experiences Mike
Michael McGrath 01:37
Look Tim, just going back, what’s your journey and what got you into AI originally, I know that originally you you had a sort of HR recruitment background, so tell us about that, and, and, and how you’ve made the switch.
Tim Hutchinson 01:52
Absolutely. I’ll try to shorten what is a relatively long story, because I’m not in the first flushes of youth. Mike. Having studied zoology originally, which was a bit of a left field educational background.
Michael McGrath 02:08
Now, for the uninitiated, including me, what’s zoology?
Tim Hutchinson 02:12
Well, take the zoo part. And is that right? I guess it’s the science of animals. Is that right? There we go.
Michael McGrath 02:18
So I presume the career options were fairly limited. And you thought, I’m perhaps going to look outside of this area
Tim Hutchinson 02:24
a little bit. At the time. So we’re talking about 1990 the environmental movement was becoming very much fashionable from a corporate perspective. And actually did a master’s in environmental management, all right, which was a highly almost vocational degree, yeah. I went from that to be an environmental management consultant, okay, with a large global construction firm, wow. And we were essentially doing so on the one hand, the construction firm was winning projects like the Hong Kong harbor crossing, yeah. And there was separation between the divisions, but we were doing impact assessment work, okay? Got it related to that, those sort of things. I did that for two or three years. It says something about me, because all while I enjoyed the learning aspects, which is really, it’s entirely a knowledge base career. I did feel at the time it was too slow. You had to really earn your stripes. It was okay. The people above me had done 20 years hard time, yeah, so I resigned, yeah. So I then I actually worked. I almost fell, as a lot of people do, into the world of recruitment with Hayes in London at the time, and frankly, absolutely loved it, yeah, and I loved it for the same reason I found the environmental work challenging, because recruitment is not slow, no, and the recruitment in the 90s, in London, it was, it was really taken off. It was like a trading floor, yeah, there are 100 of us in one room. You know, shouting, screaming, but also learning real commercial, yeah, realities, which I still lean on today, because,
Michael McGrath 04:00
I mean, I think companies started outsourcing after that recession in 1990 so it’s a huge outsourcing, get get employees off your books. And my brother built his recruitment business based on that, right on contract labor. So he had hundreds of drivers or forklift truck guys. He had literally had hundreds of them out. He was taking a margin, but, but that, have we always had that permanent recruitment where you take a cut of the first year salary? Is that always?
Tim Hutchinson 04:26
No, I think it became more popular around that time, certainly 80s into the 90s. I mean, it’s, it is, although I, I probably have a love hate relationship with recruitment. Yeah, I spent 20 years in the industry, and the last 10 years, until a couple of years ago was one of the owner directors of a tech recruitment firm in Sydney, right?
Michael McGrath 04:47
Well, it’s very mature now, so it’s kind of become a bit commoditized, hasn’t it rose? Yes, early on there was, you know, there was great margins, and it was emerging, and people were kind of using it and seeing value in it, correct? Perhaps, and and then I suppose, like a lot of things, it takes that, I think it’s called the Signet curve, you know, it kind of flattens out. And then everyone, you know, the world and his dog have figured it out, and they’re all cutting each other’s throats, I suppose, however,
Tim Hutchinson 05:12
Absolutely, however, the learnings from it. I, as I say, I still, you know, I talk to friends from Hayse days, and whatever we’re now doing, whether it’s me as the CEO of an AI business, yeah. Well, then in other walks of life, we still draw upon the first of all the work rate. We worked really hard, yeah, yeah. There was absolute, I’m going to use the word brutal, transparency over results. So the idea of measuring what matters was baked in 30 years before it became a, you know, a repeated saying.
Michael McGrath 05:47
So, I mean, what got you in to AI and what got you out of that more traditionally? Because, I mean, AI 10 years ago, you couldn’t make a living doing AI 10 years ago, couldn’t you?
Tim Hutchinson 05:55
I think, well, unless you were, I think, highly specialized and highly technical ahead of the game, pioneering the things that we now see as widely available, I think.
Michael McGrath 06:06
So, what got you into AI? And then I’m really interested in the difference between AI and a generative AI. Like, I mean, is there a difference? Because everyone’s talking about generative.
Tim Hutchinson 06:18
We’ll get to that. You give me some time to distill my thoughts. So what got me into AI, so just going back to that recruitment career towards the end of my time in recruitment, yeah, this. So this is sort of, I guess, four years ago, approximately three or four years ago, right when I exited Ember, the recruitment company, the we had noted a shift. This is when there was a real skill shortage, particularly in technical roles. And we were a tech recruitment company, right? And clients had started to talk about clients had perhaps started to open their minds, and rather than just transactionally saying, Can you find us a head of cyber security? Yeah, they recognize that. First of all, there weren’t unlimited heads of cyber security available. Yeah. And salaries were going up exponentially, yeah, because you get to a point where you’re all poaching each other’s people. Yeah. And the candidate moves, in some cases, for a 30k increment. Well, that’s like, can’t go on indefinitely. So clients started to, with our help, break down roles into the actual skills, the buckets of skills people needed, okay, and also to start to think about roles in that way. And this is the notion that has some traction now of the skills based organization now we, I guess, having hopefully entrepreneurial mindset, was exploring what that really meant and why that conversation was shifting. Yeah, and we became involved in a SaaS skills management business as part owners, the contact of mine in the UK had done the dev work and built this very rich skills taxonomy with 100,000 plus, sorry, 30,000 plus roles broken down into constituent skills, interesting with granular descriptors and map them. And which, at the time, and the world has changed again at the time, was, you know, essentially leading edge, yeah? So we started to work with companies to try and help them, using that SaaS software to understand their roles and their people by their skills. And then you start to do some of the wiz stuff, yeah, overlays of those two things, and start to shift away from the idea of linear careers, and think about a person, think about the whole person and what their capabilities might be, right now, I You may ask me how that went. Well, I now describe it as a period of heroic failure, okay, which I think we’ve only got a few few periods of them. I was about to say good periods, as long as there’s some heroic still not just failure. Why do I say that? So, first of all, we learned a huge amount, which I think is a theme, hopefully of today, but also of today’s world, where this idea of continuous learning is becoming increasingly important. Yes, so we learned a huge amount, and we also actually transacted and delivered some really awesome work. We became skills consultants to one or two government departments, right? And really sort of moved to dial in some of these areas. Where we were hamstrung, and it may got to certainly take some of the responsibility, but the tech was in some ways clunky at that time, okay? And what I mean by that is, if you bring it down to a very simple example, to skills profile an individual, yeah, they themselves, at some point, ended up sitting looking at a skills taxonomy, right? You know, it’s phenomenally dull. And I shouldn’t be so blunt, but I am so it’s phenomenally dull, and people used to get to sort of the fifth skill in the list of 100 and start to zone out, okay? And the other thing is, we’re not necessarily self aware about what our own skill sets are, yeah, at a granular level. Okay, so people would traditionally think I’m a marketing person, okay, but if you ask them to break that down into 30 skills, quite difficult to do. I mean, I mean just on that point.
Michael McGrath 10:18
I mean, marketing is completely splintered in terms of or segmented, really, hasn’t it? I mean, if you look at marketing, when I was first in business, in the late 80s, you know, there were three or four core disciplines in marketing and and now there’s, like, 35 and far fewer channels to market as well. Yeah. So you’ve got this whole digital you know, you so, I mean that that will be one area where there’s been an explosion of, you know, where that whole idea of, of, you know, buckets of skills and that the role is actually, you know, we’ve got a role here now, Matt Smith, you just met him, but, you know, Matt’s doing four jobs, and we’ve begun to break down. Okay, well, what are those four areas? Absolutely, at what stage do we have to specialize in bringing people to do that? Because, I mean, most small businesses go through this, where they have to kind of start thinking through, okay, well, you know, we can’t keep doing it. You know, people can’t keep doing everything.
Tim Hutchinson 11:14
Absolutely. And it has to be there a level of self awareness of the business as to, as to what skills are required. And yeah, and we’ll get onto this in the AI conversation, particularly because that’s a lot of what’s driving our work. But in terms of how I got into AI, one of the clients we were talking with at the time had been a successful tech entrepreneur, right? And with the advent of was it November, I’m going to say 22 with open AI and chat GPT. Essentially this, what this widespread access to generative AI tools?We probably, for about half an hour, thought it might spell the death knell of what we were doing right, and then very quickly recognized that it could well be the unlock to the things I’ve described as problematic, such as an individual looking at a list of skills, doesn’t happen anymore because of the natural language interface, right? You can have a conversation, and skills can be inferred from that now. So these are huge shifts in what’s possible, and very quickly, we’re able to roll in, at the time, our sort of vertical expertise in skills, workforce management and all those related areas, right, right, really, from from nose to tail, for want of a better phrase, from, you know, sourcing individuals for a company, onboarding them, understanding them, upskilling them, etc, that whole piece.
Michael McGrath 12:40
So that was 2022, when open AI came out with the whole kind of chapt, GPT thing?
Tim Hutchinson 12:45
Correct. So at that time, we became, you know, I think you’d agree there’s element of luck and timing to a lot of things in life, but you keep striving, you keep working hard, you keep your eyes open to opportunity, and that, that conversation, that relationship, has seen us become one of circa 15 companies in the ecosystem of a business called innovate GPT. And innovate GPT variously describes himself as a value add Gen AI venturing studio. So what they brought and bring to the party is very deep, generative AI expertise, with a lot of Microsoft alumni in the team, access to capital where necessary, and perhaps most importantly, sort of a cross pollination of ideas between the companies who are supported by innovate. So if you think of a hub and spoke model, innovate, sit at that hub, and then us and other businesses are that are the spokes.
Michael McGrath 13:01
So what was the business in 2022 you know, open AI came along, produced chat GBT went from like zero to off the chart.
Tim Hutchinson 13:59
Fastest ever uptake of technology to 100 million users, I think in three months
Michael McGrath 14:03
What was the business case coming out of that? That you spotted and said, Okay, well, what’s now needed is x.
Tim Hutchinson 14:09
I think we had people talk about falling in love with the problem. So our clients had, in their own way, many clients articulated their skills challenges, whether that related to hiring the right people, whether it related to trying to train people more quickly and more efficiently, whether it was about putting together powerful teams rather than you know, based on some actual underlying knowledge. And also there was starting to be a trend of which is really accelerating. Now, businesses are quite concerned, or very concerned, about the pace of change out there. And part of that, AI, is part of the solution, part of the problem. Frankly, yeah, because,
Michael McGrath 14:55
I mean, around that time, 2022 Yes, salaries were like, there was a huge. Yeah, the balance shifted to the employee, after being with the employer, probably for a long time, really, yes, yes. And then all of a sudden, the employees were able to start demanding. And there had been some wage stagnation, I think. I mean, we forget that, but, but there was a big catch up going on there, I think. And employers, for the first time, you know, we’re really having to face the idea that they couldn’t get people, and if they were going to get people, they’re gonna have to pay for them at levels they hadn’t even dreamt up. \
Tim Hutchinson 15:30
And although we’d moved away directly from the recruitment world, these were all recurring challenges. So I think, I think what really crystallized a business case for us is that we’d already, although it’s already shifting, we’d essentially defined the skills problem that organizations face. It’s a it’s a basket of problems, essentially. So we’d fall in love with the problem, but we’re aware, as I said, that there were challenges in solving it with with legacy tech, you know, things like a lot of the data organizations, for years in the HR space and the workforce space have been obsessed with collecting data. So there’s vast amounts of siloed employee data, but very little, very, very little insights found from it, and certainly not actionable insights. So I think, you know, we had, again, the benefit of the Innovate guys who had been building AI based tools for a decade. You know, they were some of the early developers of, for example, what they would describe as very clunky booking systems on airline websites a decade ago. So they’ve really earned their stripes. So with their benefits as well and their knowledge, we were able to recognize what a massive unlock for some of these problems, generative AI could be, because in this ability to just essentially analyze vast amounts of structured and unstructured data, find the signals in the noise for the first time, and sort of infer, as I said, either by the skills profiles or career pathways, etc, and allow users to interact without being expert in a system. It was a huge but of course, at that time, when we now look back even 18 months down, down the line, and you realize how naive you are at every stage.
Michael McGrath 17:24
I suppose, this is new, isn’t it? I mean, you know this. I mean 2002 we’re talking about, but I mean the business case. I mean, so, you know, people can download chat GBT and get information. What you’re saying the business case was that you guys were able to provide a service that allowed you to do you know, allowed you to help you know, for your customers, for your clients, you you’re able to Yeah, I see what you’re saying. Yeah, yeah. So what? How are you?
Tim Hutchinson 17:51
Let me there are obviously multiple ways to adopt AI, and we’re hopefully good people to talk about this, because I’m very proud to announce that we or to share that we’re one of four organizations granted a federal government grant under the AI adopt scheme where our goal over the next three years, under that initiative is to help 75,000 regional SMEs to adopt AI in forestry, fisheries, agriculture and clean energy.But to to sim, to simplify in terms of that AI adoption piece, you know what, what organizations are doing out there essentially is, and this is mirrored in a three step process that we now apply. First of all, there’s sort of a step one of trying to get people to a shared level of understanding. Of generative AI, get them a baseline understanding of what is this technology, what’s possible? How fast is it changing? And amidst all the hype of the noise, what? What are the practical, real world use cases that are starting to appear? We then, we then help clients with a sort of a step second step, which is, we are absolutely of the opinion that the AI initiatives should originate in the boardroom and not the server room. This is not a tech play in one sense, this is a business play. It’s about working in our instance, we work through a design thinking process with organizations. It is not a technical conversation. The goal is to have a number of sort of representatives from the organization different disciplines, and try to capture what their big hairy problems are. Or, I think I think it’s a phrase big, hairy opportunities and and then distill, distill that down into the most relevant use case to then go to step three and develop a proof of concept AI model for them. So this is, this is really very deliberate Starting off small and bite sized, because you’ve got a veryyou’ve got a technology that is evolving weekly, daily. I mean, it’s the pace of change is massive, which presents its own opportunities and challenges. And then you’ve got organizations all with their unique challenges, the solutions are not cheap, whatever that really means, so therefore you can’t, I mean, AI is fun. Everybody knows chatgpt is awesome. It’s fascinating. But you can’t, as an organization, really head off on a vanity play. You’ve got to think about what success would look like, embracing, adopting AI, how you’re going to measure that, what that return on investment looks like. So we try to work to that proof of concept, to help people understand and see the art of the possible, and to start to get some metrics prior to scaling.
Michael McGrath 21:01
Is this predominantly about about the data held by organizations and actually going to work on synthesizing that and making sense of that through through tools, or is it about more broadly, looking at at, you know, what’s, what’s available in the public domain and synthesizing that, or is it both?
Tim Hutchinson 21:24
It’s a fair question. It’s essentially both. But I think the real, the really actionable insights and the really fascinating stuff happens when you’re accessing the client’s data, because again, there are so many, so this is data that’s hell, but not really been used. There are so many. I mean, I obviously can’t mention any names, and have to be really careful. But you know, for example, you might talk. You can if you want. I mean, we won’t tell anyone. You get it in the edit. Only got six. Who are they? You can take, for example, a major retailer, who, by their own admission, have to date, been really achieving next to no insights from their sales data. If you think about a retail organization, who are I mean, what are they doing? They’re gathering data in real time. Yeah, it’s massive scale. Yeah, through every point of sale, etc.
Michael McGrath 22:19
Mind you, if there’s only two of you, and you’ve got 70% market share, I suppose you don’t, you know, it’s a bit like being the government. It’s a kind of tax on So, I mean, the, I mean, necessity is a mother of invention, isn’t it? And generally, we, you know, we, it’s the problems that drive the innovation and solutions, but it’s good that they’re waking up to that.
Tim Hutchinson 22:38
But, well, let me give you an example then, which is probably not probably would be a combination of those two broad sources of data you touch on, a lot of our work at Skills GPT is really trying to find opportunity and disruption for organizations, particularly around their workforce. Now what I mean by that is we’ve got some fairly massive disruptions happening. They are macro disruptions. So, AI, the emergence of AI, of course, people are concerned about what that means to jobs.
Michael McGrath 23:15
So give an example of some of the disruptions that you’re seeing. So that’s binary, really, you you’re not talki
Tim Hutchinson 23:18
I’ll give you an example from a conversation yesterday where, yeah, a client in the energy sector, because a lot of our work is, is, is moving to sectors such as under transition. So the transition to clean energy, yeah, from Legacy sources, okay, is a brilliant example of workforce disruption, okay? Because you’ve got, fundamentally, you’ve got legacy jobs rolling off in simple terms, from, you know, the coal, gas industries, yeah, and you’ve got an increasing demand for people with knowledge and skills around renewables, okay, you know, making connections to solar, to work, etc. well, so that that’s, that’s a big external disruption that’s happening from, I mean, from a tech perspective. So sorry, I was going to explain even in that instance. So there’s, there’s that sort of macro disruption, yeah, and then they so, there are challenges there in terms of finding the right staff. They don’t actually exist at scale with those renewables experience. Okay? So they started to look at efficiencies in the existing workforce as a starting point. Yeah, even if you can find one or 2% efficiencies, it all helps. Okay? So, you know, at one point in time recently, they’re looking for more people with scheduling, rostering, planning experience, yeah, but two or three months down the track, recognize that some of the AI tools are able to perform that task, right? So, as opposed to the needing people with rostering experience. You pivot. And what you actually need is people with the ability to right size those AI rostering models, right so that and this, this, these are they different kinds of people. So they’re used talking about technical people versus, you know, project management type people, in simple terms and this. And this is where you start to think, Well, what actually is, where does knowledge fit for people? Is it? Is it functional knowledge? Is it technical knowledge? Yeah, and this is, there is no answer to it, because this is a rapidly moving piece. We’re not building product at this stage. No, we’re not building out of the box SaaS product, because in most cases, we’re trying to find a highly effective point solution for a priority problem around workforce, and then to develop from there.
Michael McGrath 25:52
Look, I mean, I suppose the real challenge is that, I mean, you know, most of probably listen to this podcast are small business owners, right? About 40% of them are apparently. So there’s, you know, hundreds of small businesses that are going, you know, they hear AI, they get the fin review, you know, that they read articles like, will AI be a bust?
Tim Hutchinson 26:15
And and, surely not the binary, the binary press, yeah.
Michael McGrath 26:19
And so we’re looking at, okay, how do I harness this? How what? What’s in it? For me, because, you know, you think about woolworths or coles and all their data, and you go, Okay, well, you know, you can think, you can play the long game. You can, you can invest in this. There’s such a business case there. I mean, you can already see from the amount of data they must have, if you could start to do something with that dynamically and get insights.
Tim Hutchinson 26:44
And the proven ROI is already massive, yeah, so it’s off the chart, yes, but was it?
Michael McGrath 26:51
You know, your small business, they employ 50 people, and they’re trying to think through, okay, well, how do we harness this thing, other than we’ve got chat GPT on the desk, or Microsoft co pilot, and we’re just plugging in questions, yeah?
Tim Hutchinson 27:05
Well, yeah, but, but even that, actually, I mean even even that part. So you know, if you think about chat GPT, and I’m sure listeners are using it for various tasks at home and, dare I say, at work, but with the security concerns, very quickly, small organizations started to get their own sort of secure instance through 365, so they therefore can have that conversation with chat GPT securely. And it’s a good point, because actually organizations you start to the people who efficiently are finding good use for it, are the workers. So if you actually ask people what they’re using it for, and have an open, honest conversation, that’s where you learn the insights. And people will be using it for report writing. They’ll be using it for structuring reports. They’ll be using it for planning their week. Every possible sort of entry level use, if you like. But I think what’s happened is, I think you mentioned the newspaper there. You know, we have this strange binary press where you have to go through a hype cycle, don’t you? Where, first of all, it’s, it’s incredible. It’s, it’s, it’s going to solve all the problems in the world, and then a week later, it’s going to, going to be the end of all work. Yeah, all jobs are gone to let that pass. And then, you know, now we’re at a stage where companies are trying to or beginning to understand what’s possible, yeah, and trying to find these practical solutions. However, you know, there is, I mentioned cost earlier, and actually, for SMEs, there is a cost factor, yeah, and in many cases, to, you know, to have a we get a lot of requests from people who, I think I’ll think of one very close to home. The other week, a recruitment company who said to us, Hey, we’ve, of course, got a CRM with 100,000 candidates on it. Could we, you know, put put that in their terminology, put that into AI and find interesting things right now. The answer is, yes, absolutely, okay. Does it come at a cost? Yes, absolutely, yeah. And because of this pace of change in the world, you can’t, in good conscience, stitch somebody a solution together and leave it with them. No, you actually need to then, sort of offer a managed service, yeah, to continually help that model develop, because it’s a continuous improvement thing. You know, there’s, there is a difference between, um, so I’d say two things broadly, and they’re not, they’re not their objective, hopefully not in any selfish interest one is with AI, the best thing to do is to get started. Now by that, let me clarify. I don’t mean get started. Start throwing money at the problem. I mean start learning about it. Start reading about it, start trying to at least understand what it is. If anyone wanted to reach out. LinkedIn, afterwards I’ve, we’ve got various resources that point to, you know, a nice, a nice checklist of 20 resources they can go to for free to start that learning journey, yeah, because I think you need to understand it first of all, yeah, you know. And then, and then the other piece is probably to to talk, to talk, to talk to existing staff, because people will be using chat GPT, and rather than pretending they’re not, from a security perspective.
Michael McGrath 30:29
So tell me about the security risk, right? So you know someone in a small business, so there’s 20 staff, and half a dozen of them start, you know they you know that they’re using chat GBT, and they haven’t told anyone. So where are the risks for the business there?
Tim Hutchinson 30:45
Well, I think, I mean, the the risk is that you are essentially sharing information publicly. So very I remember quite early on we had, and it feels like a lifetime ago, but it but it was a year ago, a CFO was stood up in a meeting we were in and sort of, um, laughing about it, said, Oh, I’ve uploaded the annual accounts, and I’ve started to get some insights. Okay, it’s really, really not a good idea. Wow. Now, generative AI chat, GPT is not a search engine, no. So as such, that packet of information, that set of accounts, isn’t sitting there to be accessed in its entirety. However, it’s something of an unknown as to what aspects are. So absolutely you shouldn’t be sharing sensitive information. But as we all know, straight censorship is not an answer to anything. So I think you need a discussion with staff and really understand, yeah, okay, because that’s again going to that point about it originates in the boardroom, not the server room. Take the workforce as a proxy for the boardroom. Find out what people are using it for. Find out where the efficiencies are. And the other thing I’d say I quite like this is I’ve got a colleague, Ray Fleming, who tries to explain generative AI in simple terms and says, treat it as an intern. Okay? I quite like it, because if you think about an intern, you get the best out of them by giving them the best direction, simple, structured direction. You find value in the work, typically quickly, but you typically, or should check the work. And that’s very much the same with generative AI. You know, there are phenomenal insights, but I think you do have to also take it with a pinch of salt, because of hallucinations people will have heard of and make certain that you’re either applying your own critical thinking, or the guys who built the models for you have thought about what, how the information that surfaced is is verified and against what.
Michael McGrath 32:56
So can you give us some examples, Tim of of you know, good use cases where, you know, the the the ability to do some of the things that you’ve been talking about has really helped a business, you know, transform
Tim Hutchinson 33:10
I can. This is where I have to be careful, because some of the detail is, is, it’s very specific. I’m going to give you an example that that was a very early use case that we prototyped, because I think it, in one sense, it’s very simple, and in the other sense it’s also it’s very much a people first example, which I like. I don’t want to give deeply technical examples. So we were working with an organization who are essentially helping disadvantaged job seekers to secure work so disadvantage for a range of reasons, but sometimes mental health challenges, socioeconomic challenges, etc, now, and it talked about A large organization now, the challenge they had is that despite working hard and wanting to do the best by their clients, it often ended up as a somewhat binary matching exercise. So if somebody had done some cleaning work in the past, they would look through their opportunities and find a cleaning job. It achieves something. It also misses out on that point I made about other things they could do, yeah, yeah. What do they aspire to? What are their motivations? Okay, what else are they capable of? Now, again, as I said earlier, that’s a cohort of people who perhaps find it harder than others, yeah, to self select and understand what their aspirations or their skills are. Yeah, now we then applied generative AI to both sides of that, the supply and the demand piece of that problem. So on the demand side, the job seeker, we’re able to build something called role narrator, okay, so. Which essentially they had a conversation, an actual conversation, not even a typed conversation based on a series of questions, which really focused on just what they enjoy in life and what work they’ve done and what they enjoyed about that work. Okay, no pointed questions around open questions really open and express themselves. Yeah. Now from that, you can very effectively infer what somebody’s skills are, but perhaps as importantly or more importantly, what are their motivations, what are their aspirations, what are their fears about work, whole range of things. Now, if you do that as well, not so much conversation, but if you start to look at the roles on the supply side and think about them again, beyond the job title, okay, and what the attributes are, you start to find adjacencies, yes. And you know there were some very, very specific examples where you know somebody in that instance, who was aspergic had made a comment around preferring to work alone. And it seems really simple, but they’d actually been overlooked for a whole range of cleaning roles. In the cleaning instance, because, of course, there’s people in the offices, etc. But then it started to think about some of the skill sets, some of the skills, yeah, around handling equipment or handling certain chemicals, whatever it might have been. And, you know, a role pops up, which I think from memory was It was certainly a night shift role, which might seem obvious, but people don’t make these connections at scale, yeah, normally, and it was in a completely different discipline.
Michael McGrath 33:56
No, no, that’s good. And, you know, I really like that example. So ostensibly, you’ve got these, you know, you’re breaking down a role from a time to a bucket of skills, and then you’re getting much more insight into the candidates and what it is that may have done in the past, or what they could do, what they like, what they don’t like, and then you’re trying to intuitively put that together in a way that it wasn’t being done before, certainly. Because, I mean, I mean, what little I know about recruitment is it’s so easy to get pigeonholed in any recruitment Never mind. Yeah, absolutely, you know. And you know, often, you know, we’ll talk to people who really want to make a move it’s in a traditional recruitment sense that’s very difficult.
Tim Hutchinson 37:24
Oh, absolutely, yeah, absolutely, if not impossible, you know. And perhaps, I mean, I’ve given a recruitment example, essentially, and I really, I need to make it clear that we’ve moved far beyond recruitment, and, you know, broadly talking about workforce. But even a recent example,you know, if you take any of these industries that are transforming rapidly, and I will mention the energy one again, because it’s really the largest global initiative of all time, the shift to clean energy now, when you talk to clients in that space, even forget the internal challenges they have around workforce. They’re very aware that they don’t necessarily know what they don’t know about the sector they work in. And what I mean by that, there’s vast amounts of information out there about battery energy storage systems, about solar projects, all of this stuff you can now assimilate that information at scale and start to make you know, as is, insights around demand and project demand, and hence workforce demand, but also you can start to make predictive ideas about where is our industry heading. What might that mean in terms of the skills required, headcount required, the costs, and what are the levers that are going to impact that? Now, what you find is, when you start to look, you start to see things, and you know, in that instance, you have a new challenge evolves, which is the biggest lever in that external piece is regulatory landscape, which you can’t really necessarily dictate. So then you have to look at, what can we again, what do we measure to allow us to plan ahead? And a lot of organizations are remarkably in the dark currently.
Michael McGrath 39:17
I mean, there’s a lot of talk about AI taking out jobs, and you know, the workforce going to be dramatically affected. And you know, those predictions were being made in the in the late 80s, with the advent of the computer, really. I mean, there was a lot of talk about, you know, I mean, when I left school, there’s 10% unemployment if you’re under 25 that was about 25% and so there was a lot of talk about computers are going to take over, and there’s going to be no jobs. And yet, we haven’t seen anything like that, have we, really. So, I mean, AI is now talking about that as well, but it’s going to take it now. I mean, is that going to happen? Or, in fact, are we just going to see a pivoting, really? And.And a redistribution of of work,
Tim Hutchinson 40:03
Honestly, I think it’s hard to predict, because you’ll hear every variation in the answer, yeah. I mean, absolutely categorically, certain jobs will disappear, yeah, and other jobs will significantly change in terms of their profile, right? But does that lead to wholesale loss of people? I don’t think so, because, probably broadly, for two reasons. One is that that pace of change just does not impact that quickly. People are no there’s inertia in the system. People organizations are slow to adapt. Yeah, perhaps rightly so.
Michael McGrath 40:38
I mean, and you wonder where the triggers are. I mean, if you take, I mean, when you get to my age, you can look back a bit. So the if you look at websites, have been spoofed in the 90s as the answer to everything, yes. And you know, I kept, you know, being convinced that I had to look at it, and then I’d, I’d ever go online, and then, you know, I’d see the dialog, remember the old dialog? And it would take like, five minutes to load a page, yes, and it was like a brochure online. And I remember thinking, what a lot of fuss about nothing. And then everyone was going on and on about that. And literally from the early 90s right the way through 2000 and then it the whole penny dropped when the iPhone appeared in 2008 and all of a sudden we went to commerce, yes. And speed and convenience, yes. And that was a catalyst for a massive, I mean, look at the amount of commerce done online now, absolutely.
Tim Hutchinson 41:29
So look at what that’s led to in terms of allied industry, exactly. So you wanted housing, logistics, distribution, yeah.
Michael McGrath 41:36
So what are the triggers, you know, for AI, maybe you know what, what’s going to make the chain the wholesale change there in terms of adoption and utilization. It’s hard to know, isn’t it?
Tim Hutchinson 41:45
Honestly, I think it’s very hard to predict. I think, um, I mean in practical terms, I would say to organizations again, this is not going anywhere.
Michael McGrath 41:56
So I think so get hold of it. Educate yourself. Hold of it. Understand, trialing stuff slowly, methodically. You know, what can we do Well, either the fees go down or they keep hold of the fee. I mean, I mean, that’s the thing is, does that get anywhere?
Tim Hutchinson 42:06
At an individual level, the same this, this sort of idea of, you know, lifelong learning. You know, it’s an, it’s an oft repeated phrase now, but that you’re not going to lose your job to AI. You’re going to lose your job to somebody using AI. And, you know, people are very it’s, again, go back to that intern idea. It’s almost as if each employee has an intern supporting them. Now, if you get that right, there are just massive efficiencies and upside now, whether they’re, I mean, you can, it’s hard to come up with examples on the fly, but you can look at certain industries where there will be wholesale change. But actually, you talk to people in those industries, they don’t necessarily, they can’t necessarily predict where that will end. So take something like the legal profession, for example. You’ll it. I was with a group of lawyers the other day who both have a they have a legacy law firm, traditional, successful law firm, and also for moving into the generative AI space. Now they’ve almost done a parallel run, an AB split testing on work that they do repeatedly. And I think roughly they’d said it would take a team of four people three weeks.Traditionally, they’ve now got that down to 20 minutes with an AI model. Now clearly that’s a fundamental change. What does that mean? The fees probably remain relatively high and then erode as it becomes more apparent that that that’s the case. But for the but for the lawyers, um, you know, they’ve gained back a lot of time there. So is there, you know, the the simple answer is that there’s value add work they can be doing. I did hear I’ve got to be careful here as well. So I’ll edit this a little bit very early on again. And I’m talking a couple of years ago, I was talking to a CIO of a fashion business who was very head of the generative AI curve, and partly because their CEO was essentially from that world, the Chief Information Officer, right? Yeah. So the CEO is from an AI world previously, and then the CIO, therefore had that influence early on, the CIO quite quite a bit of a character, had presented to the design team and others around generative AI and in real time, typed in some of their design cues as an organization, and asked it to produce an item that they typically produce, yeah, and it did, so, okay. And it was arguably, well, certainly was very similar to what they produced previously designed, and I’m being careful with my detail, but actually, many ways, was better. And he deliberately left them in. Sort of looking glum for a while, as if, right? Well, that’s us done. And then a bit like in a reality show, where they start, they then turn up the turn up the color, and turn up the rousing music in Grand Designs or something. He does the uplifting part where he says, hey, you know that thing on our sort of to do list that we’ve all wanted to get to, which is looking at a new sustainable material to replace leather. Now, we can do it. We’ve never got to it, have we? We could be doing this. So there’s this shift of adding value, adding value, hopefully doing the work we’re more interested in. Yeah, of course. Look, I’m an optimist at heart, and I think that’s an exciting future. Yeah. Will there be collateral damage along the way? Of course. But I heard an example the other day as well from image and computers in the 80s. The chap I saw presenting was talking about the motor car at the turn of the century, you know, whatever it was. You know, late 1898, 1900 and, you know, there was something like 30 or 50,000 people working in the horse stabling industry in London, yeah, um, now the motor car came along. Yeah. There were fewer horses. Yeah. Now what happened? That happened over a period of time, blacksmith started helping in the car industry, yes, etc. So, you know, look, I’m not the person to predict, and I think anybody who does
Michael McGrath 46:27
Look, who are the winners here? I mean, if you look at open, AI, right? I heard the other day they started out as not for profit, but I heard they flagged the other day they’re going to a profit model, right? So who’s going to be the winners here, right, in terms of the economic model, and who’s going to, kind of, you know, the big, are the big tech guys going to own this space, and we’re all going to get the scraps, or, in fact, is it? Is it going to be a genuine democratization of publicly available information?
Tim Hutchinson 46:54
It’s possibly a bit of both. It sounds like a fudge, but bear and bear this in mind. So in so many areas of technology, it’s technology has been pioneered by the small niche players and subsequently acquired by the big tech. Yeah, this is one of the first instances where it’s the it’s the exact opposite. So almost overnight, big tech have released this phenomenally powerful technology, and it’s now the smaller players who are trying to work out what to do with it, yeah, and how to commercialize it best. Now where that will will lead to, my best guess is, as in a lot of industries, you will end up with people with highly specialized, niche capability that’s highly effective, right? So the guys who are pioneering, for example, you know, looking looking at radiography or cancer scans and doing very niche work there very effectively in terms of identifying cancers. Of course, there’s commercial upside in that, whilst doing good work. But you’ll also have, presumably, a handful of big players who win in the space. Yeah. So if it you know, and and again, another cliche, the idea of selling shovels to gold miners. I mean, look at Nvidia and their chips and their share price. Well, they’re so well placed to to service the entire industry, regardless of what’s been, what the use cases are, yeah, interesting. I mean, I think in our world, it’s a very good point as sort of, you know, founder of a scaling, generative AI business, we have to think about these where our commercial future is, yeah, and in some ways, that’s you certainly can’t determine that hard and fast from day one, no. So I think, I think what we try to do amidst the noise is to pick out the things that are tangible. So, for example, that grant wind delivering AI, yes, helping 75,000 organizations adopt AI. And if you want to see more about that, you can check out arain.com.au Australian regional AI network. That for us, is an enormous opportunity, because amidst all the noise and all the hyper generative AI, that’s a vote of confidence in us, and it’s a tangible roadmap to helping organizations at scale. Wow, some of these initiatives may ultimately lead into, you know, repeatable use cases that then lead into product. And we’re almost doing some of the market testing, you know, with with a with a large cohort, if you like, what we’re very aware of, we cannot be all things to all people. So I think it’s one of those businesses. Certainly for us, we’re running our business with no sacred cows, as they say, yes. So I think the best thing we can do as a leadership team is is put everything on the table every quarter, yeah, and determine, again, to measure what matters, determine our direction. Yeah. And, you know, put, put our, put our efforts into those things that have future.
Michael McGrath 50:07
In some ways, we’re, we’re back to the beginning. We’re applying timeless principles to, you know, to technology, hard work, measurement, results, you know, figuring out how something can be harnessed to be useful and constructive and positive and absolutely remembering people in human in the loop, critical, yeah, and there are downsides everywhere, like there is with everything, including the internet. But, but if we, you know, if we can keep, you know, if we can keep positive and constructive, we can, we can, perhaps, you know, carve out a better future certainly been the the history of mankind really, is that we, we have moved, found a way to move forward really absolutely.
Tim Hutchinson 50:47
And I would honestly say to people, I’m probably repeating myself, but I think now, if you’re an organization wondering about AI, interested in AI, terrified by a whatever it might be, don’t worry about the technology for the moment, other than,I think, accepting that it’s here to stay and will be ultimately have a bigger impact, I think, than the internet. Yeah, but don’t worry about the tech. Park that bit of conversation and think about your business, and think about those areas where you deem there to be really significant opportunity or that have been the bottlenecks to growth. Now,despite running an AI business, I’m repeating another phrase you hear a lot. Just because you have a very large AI hammer doesn’t mean that everything is an AI problem, right? So it’s not the solution to everything, no, but the starting point is to identify the business challenge and to work back from there and determine whether and to what extent AI might produce a new unlock to that problem, right? And that’s where you then start to actually find tangible benefit, or determine that at this stage, or for your business, it’s not appropriate.
Michael McGrath 52:04
Yeah, get it. That’s interesting. Look fascinating. Tim. And on the subject of lifelong learning, I mean that idea that we’re, you know, we’ve all got to become kind of lifelong learners and students, I think. And now, with the democratization of intelligence and information, really, you know, via the internet, we’ve got so much more available to I wasn’t going to
Tim Hutchinson 52:23
Sorry to interrupt Mike. I was reflecting on something walking down here, which is, in some in some ways, as a, as a 50 something chap, I’m a, I’m a bit of an example of this idea of lifelong learning, and I kind of reflect the the pace of change in the world. So, you know, obviously, studied early on in life. I imagine I learned a few things in the interim. However, I kind of got busy with work. It’s in this last three or four year period where I’ve massively embraced the idea of learning, and I am loving it, because my brain feels just alive and but I think a lot of people suffer from imposter syndrome, don’t they? Which? And they sort of feel they can’t enter a new world, or they’re not worthy of it. I heard a funny enough, a stand up comedian, the other day saying these people don’t have imposter syndrome. What they what they don’t realize is they’re suffering from a healthy dose of self awareness. So, in fact, a lot we don’t know. We don’t know a lot of stuff. And I think it perhaps comes with age or something where I no longer care whether I know what I need to know in a situation, because most people don’t know everything, and there’s a lot what I’m getting to I think, is saying this pace of change in the world is in some ways great, because nobody’s got all the answers. So I’m in meetings where I’ve gone back to basics, where I’m applying perhaps recruitment skills. I ask questions in a humble, unafraid manner. Might be a bloody basic question. I have no idea, but I asked the question, and I listened to the answer, and I think in that, and that’s a good way of looking at looking at AI, which is try and understand it, try and explore and, you know, get involved, because people don’t have all the answers. You know, if something’s released, you know, constant new releases on a weekly basis, you can know as much as anybody else in a week’s time.
Michael McGrath 54:18
Yeah, look, Tim, thank you for that look. It’s a fascinating world ahead of us, and we certainly got to be alive and awake, haven’t we, and I’m very grateful for you coming in today and sharing with us your insights. We’ll put your details in the show notes. I appreciate the opportunity, Mike, and we wish you well at skills GPT, thank you very much. Good luck.
Tim Hutchinson 54:39
Thank you very much. Take care. Bye.
Michael McGrath 54:44
So there you have it, generative AI. Thanks to Tim for helping unpack how AI is being developed at the practical level. And whilst the future is always uncertain, what’s not uncertain is that AI is going to be part of it. That’s for sure, if you want to chat with Tim at Skills GPT, the details are in the shadows now, a final shout out to Oasis partners, our generous sponsors of this podcast, Oasis help the shareholders of private companies wishing to exit strategically. So if you want to know more about what they do, talk to Don hunter and the CEO of Oasis. So if you enjoyed this episode, please tell your friends, and if you’re feeling generous, leave a like until next time. Take care you.
Link to previous episodes: https://www.oasispartners.com.au/podcast/nick-armstrong-unlocking-the-power-of-ai/